Data Modelling and Predicting the Future
All data models are wrong.
It might seem bold to say this but it’s because, fundamentally, that’s a truth that needs to be accepted.
Whilst business intelligence analytics have become a hugely valuable area, what’s important to understand is not that the data is always accurate at perfectly predicting the future, but instead, that some models are useful.
If you think about it, E = mc2 doesn’t actually mean anything. Instead it very closely approximates how matter behaves, therefore it seems favourable to us.
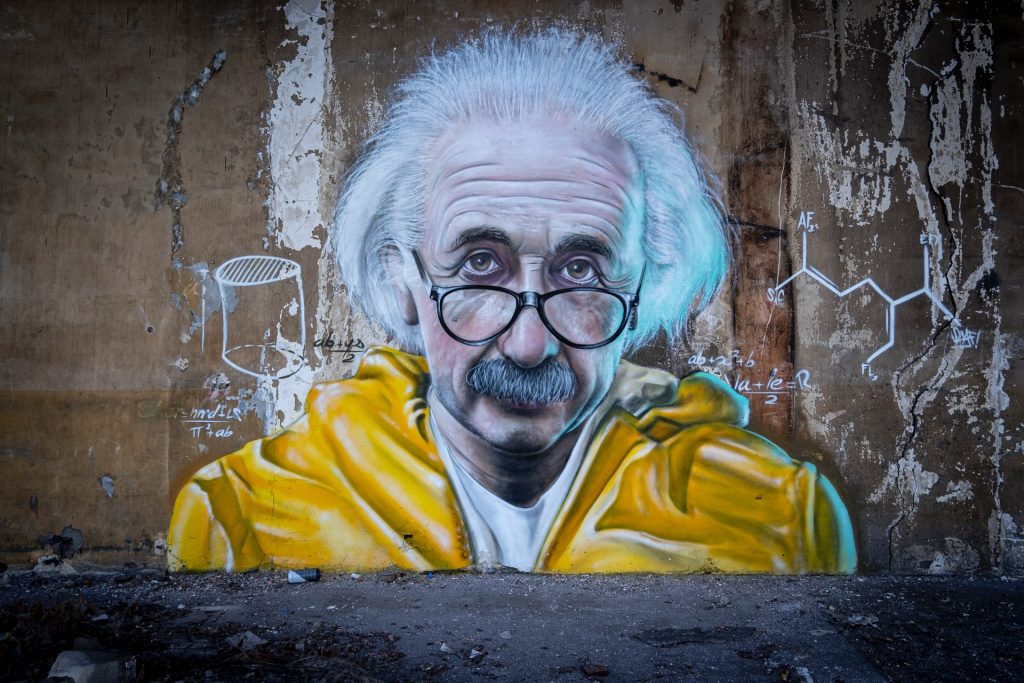
Photo by Taton Moïse on Unsplash
In exactly the same way, if you create a model that tries to predict a future outcome, it’s not going to get it right every time. However, it gets it close enough to help you make those all-important business decisions and projections.
That’s what data modelling is about. It concerns how you can understand your data better and how automated processes deal with a much greater volume – allowing you to be more accurate and swifter in your decision-making than with human operators.
To adjust the thinking around data via machine learning (ML) and artificial intelligence (AI), you need to truly get to the bottom of what you are trying to predict – and therefore understand.
Ask yourself these questions:
- What data do you think will be important?
- Do you already collect this data?
- If not, why not?
Knowing this could be the hidden gem in developing your organisation’s future and will help you to find ground-breaking results. Remember, you don’t have to be a data scientist to get value from your data. Just having easy access to such intelligence will set your business apart from the competition.
To help transform your thinking, let’s start by debunking a few myths…
Myth 1: An analytics model will solve ALL your problems
No, it won’t.
It still needs you to advise on the data that you’re already collecting. However, a model will provide a pathway to open up your data, and identify opportunities so you can foresee what’s likely to happen.
It’s vital to do the intervention quickly and know the difference between causation (the human element) and correlation (the machine’s input). Ultimately, one doesn’t cause the other because there is no mathematical way of defining the former, only the latter.
Putting this into an example…
Think about students who visit the library in their school, college or university a lot. Does that indicate that they’re likely to stay in college? Perhaps.
But what that doesn’t mean is you should make a rule that demands every single student has to go to the library – because you won’t get the same outcome.
ML – Machine Learning – is a helper to you, not the other way around. With any kind of automation, it should allow you to make clear choices quickly but still enable you – the decision-maker – to focus on the trickier, more humanistic elements.
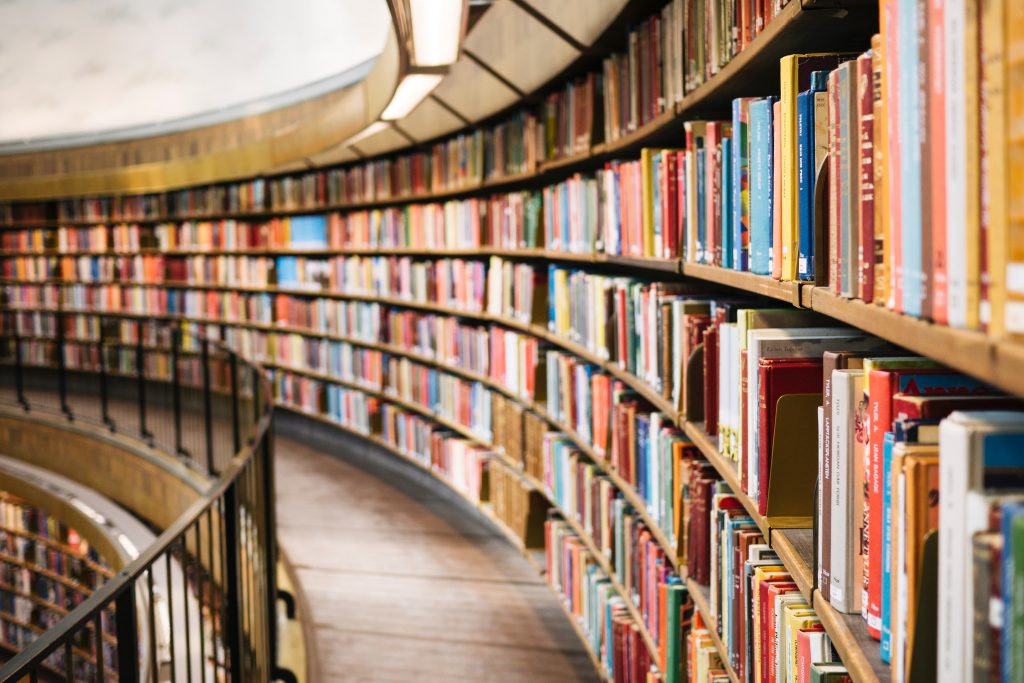
Photo by Susan Yin on Unsplash
Myth 2: AI can instantly automate your lives – and replace you
Don’t worry, you’re safe!
Machines won’t take your jobs. If anything, they should enhance your roles.
That’s because savvy tools are there to provide insights that are not emotionally driven. They’re using mathematics to drill down the data and are in fact taking away the more laborious, mundane tasks so you can prioritise the matters that automation can’t naturally solve.
You should trust in the machines and work collaboratively. ML must engage operators so that they understand the decisions it is making, and therefore act swiftly.
Think of ‘Hawk-Eye’ technology in tennis. It’s used to limit emotive moments, shutting down any questionable decisions with critical evidence to back up the umpire’s call.
Every single person watching can see exactly how the decision has been made by the machine too, which introduces a greater level of fairness and trust that wasn’t there before.
Line judges provide the human element, but it’s the machine that augments their decisions.
Ultimately, you are a vital element for the success of your analytics model because you’re the observer who knows when you need to intervene – and when not to.

Photo by Mudassir Ali on Unsplash
Myth 3: Your data needs to be perfect
Again, this is a firm ‘no it doesn’t’ answer.
Data will never be perfect. Instead, adjust your thinking by taking what you have at the moment, looking at it and deciding where to apply your focus so that you can gather more quality detail in future.
We’ve demonstrated before that with just a few, simple data points, you can quickly gain valuable insights when you run it through powerful business intelligence tools (see: How I used BI software to predict films I'd love).
Your analytics model should be able to extract information very quickly. Yes, it might tell you that your insight isn’t good enough, but equally, it could provide you with intelligence that you didn’t previously know.
Data is critical across all sectors. For example, if you work in a school, it can help you to analyse student retention and welfare so that you can predict which individuals are most likely to drop out.
If you’re part of local government, it can reduce public sector housing costs via predictive maintenance regimes.
Perhaps it can be plugged in to assist in how a marketing department projects customers at risk of leaving – and therefore drives the organisation to automate incentive schemes which encourage them to stay?
Alternatively, healthcare specialists can forecast patients most likely to miss their appointment – and intervene while time is on their side.
Starting your analytics and AI journey sooner rather than later can help you to understand what data you need and how to evolve it so you can make these all-important business decisions swifter and smarter.
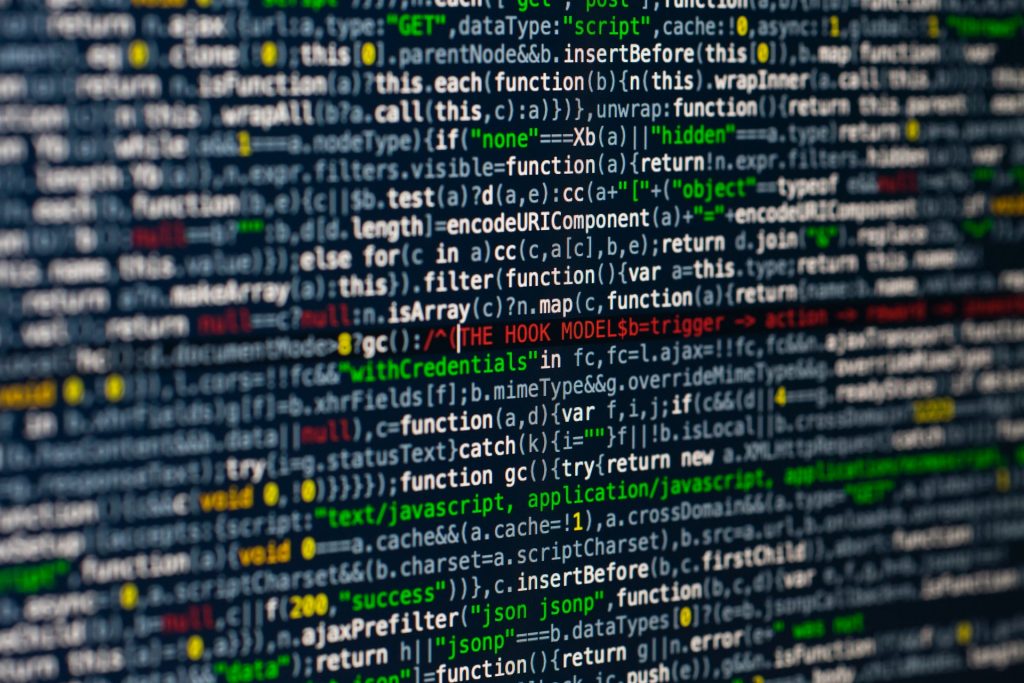
Photo by Markus Spiske on Unsplash
A recap of the business analytics rules
When it’s done right, ML should always be repeatable, justifiable, and understandable. It should enable you to examine decisions quickly and remove a huge element of bias. So, remember:
Rule number 1
Don’t dismiss the data. Try, test, and keep remodelling.
Rule number 2
Provide additional information to help improve your data and clarify previous outcomes.
Rule number 3
Before you tap into the world of analytics, know exactly what you want to get out of your data – and why you need it to provide you with clearer insights.
Ken Miller is the Cofounder and Product Director at Panintelligence. If you'd like to learn more about how analytics tools can benefit your business - book a free pi demo here.